Impact statements
The development of urban water infrastructure (UWI) has been driven by the evolving needs of customers, society and the environment but now faces a number of profound challenges such as climate change, ageing assets, stringent regulations, increasing demands and inadequate investment. Artificial Intelligence (AI), a widely recognised disruptive technology, is shaping an increasing range of sectors such as healthcare and banking, however, there is a critical knowledge gap in understanding how AI will enhance the performance of water systems and revolutionise the future development of water systems in practice. This review provides an analysis of how AI could be aligned to support the five development pathways: decentralisation, circular economy, greening, decarbonisation and automation. This shows profound opportunities of AI application for the water sector in terms of how interventions should be developed and incorporated into the existing infrastructure system using AI-based methods and tools. In the meantime, practical implications and barriers arising from application of AI-based tools should be fully recognised and responsibly managed considering the following four dimensions: cyber-physical infrastructure, institutional governance, social-economic systems and technological development. Examples include data privacy, ethical and legal concerns, fear of job loss, lack of adequate skills, risks and unintended consequences from failure of AI systems. It is envisioned that trustworthy AI technologies will play a pivotal role in moving towards reliable, resilient and sustainable UWI systems.
Introduction
Urban water infrastructure (UWI) is fundamental in providing essential water and wastewater services and supporting the prosperity of cities. It has been evolving gradually to meet the increasing needs of society, ranging from water supply security, public health, flood management, social amenity, environmental protection, resources efficiency, ecological integrity and climate resilience (Brown et al., Reference Brown, Keath and Wong2009). However, UWI is now facing a wide range of chronic and acute threats such as increasing demands, stringent regulations, ageing, lack of investment, climate change and extreme weather events (Butler et al., Reference Butler, Ward, Sweetapple, Astaraie-Imani, Diao, Farmani and Fu2017). There is an urgent need to develop resilient and sustainable UWI which can maintain and improve service levels under future uncertainties and threats. A key approach to tackling these threats is provided through capitalising on the advances in information and communications technology (Lund et al., Reference Lund, Borup, Madsen, Mark, Arnbjerg-Nielsen and Mikkelsen2019) and AI.
Digitalisation of UWI systems has come a long way and now lies at the heart of the transformation towards the fourth revolution in the water sector, so called Water 4.0 (IWA, 2019; Daniel et al., Reference Daniel, Ajami, Castelletti, Savic, Stewart and Cominola2023; Rapp et al., Reference Rapp, Capener and Sowby2023). The advances in digital technologies enable the construction of the “cyber system” on top of the physical infrastructure system, which provides the following main functions: sensing and instrumentation, communications and networking, data management, cyber-security control and decision support. Supervisory control and data acquisition (SCADA) systems are widely adopted in water distribution systems, water treatment works and wastewater treatment plants (WWTPs) to provide real-time and in-situ monitoring of pressure, flow, water level and water quality indicators and remote control of actuators (e.g., pumps and valves). This was mainly driven by the pursuit of efficiency by water utilities and technological innovations in developing low-cost, low-maintenance and high-reliability sensors (Yuan et al., Reference Yuan, Olsson, Cardell-Oliver, van Schagen, Marchi, Deletic, Urich, Rauch, Liu and Jiang2019; Garrido-Baserba et al., Reference Garrido-Baserba, Corominas, Cortés, Rosso and Poch2020). However, systematic understanding of how UWI system performance can be enhanced through digitalisation is limited.
AI technologies have long been applied to UWI planning and management, however, there is a critical research gap in understanding how AI enhances the performance of UWI systems. Although there is no consensus on a single definition of AI, here it refers to the development of computer systems or machines to perform tasks that would normally require human intelligence such as learning, problem-solving, decision-making and perception (Xu et al., Reference Xu, Liu, Cao, Huang, Liu, Qian, Liu, Wu, Dong, Qiu, Qiu, Hua, Su, Wu, Xu, Han, Fu, Yin, Liu, Roepman, Dietmann, Virta, Kengara, Zhang, Zhang, Zhao, Dai, Yang, Lan, Luo, Liu, An, Zhang, He, Cong, Liu, Zhang, Lewis, Tiedje, Wang, An, Wang, Zhang, Huang, Lu, Cai, Wang and Zhang2021a; Richards et al., Reference Richards, Tzachor, Avin and Fenner2023). A broad suite of AI technologies have been developed for specific tasks such as system simulation, optimisation and prediction. Examples include evolutionary algorithms for optimal design and operation problems of water systems (Nicklow et al., Reference Nicklow, Reed, Savic, Dessalegne, Harrell, Chan-Hilton, Karamouz, Minsker, Ostfeld, Singh and Zechman2010; Li et al., Reference Li, Li, Chang, Sharma and Yuan2022a) and artificial neutral networks for flow and water quality prediction and anomaly detection (Fu et al., Reference Fu, Khu and Butler2010; Rodriguez-Perez et al., Reference Rodriguez-Perez, Leigh, Liquet, Kermorvant, Peterson, Sous and Mengersen2020). Machine learning, a subset of AI, allows systems to learn directly from data, examples and experience without pre-defined rules and is currently at the heart of AI implementation (The Royal Society, 2017) and has been applied to understand complex water systems and discover new insights from various data sets (Eggimann et al., Reference Eggimann, Mutzner, Wani, Schneider, Spuhler, Moy De Vitry, Beutler and Maurer2017; Liu et al., Reference Liu, Savic and Fu2023). The latest deep learning technology, which is a subset of machine-learning responsible for the current rise of AI, enable automatic feature extraction from raw data and have been used in a diverse range of urban water management problems, predominantly covering anomaly detection, prediction, asset assessment, operation, and planning and maintenance (Fu et al., Reference Fu, Jin, Sun, Yuan and Butler2022). However, AI applications in the water sector are based on a piecemeal approach and the insights gained from various technologies are not joined together to advance our understanding of how AI will revolutionise the development of UWI systems of the future.
This review addresses a key question: what is the fundamental role of AI in the development of resilient and sustainable cyber-physical UWI? It aims to provide a holistic synthesis of how AI could be aligned to support the development of UWI systems of the future. We first explore the ways that AI can enhance the performance of UWI systems in terms of three key aspects – reliability, resilience and sustainability, and then develop a routemap of how AI will shape the future development of UWI towards greater resilience and sustainability. This review shows that AI is indispensable in the digital transformation of the water sector and should be aligned to underpin five pathways -decentralisation, circular economy, greening, decarbonisation and automation (see Section 3) - through which the current UWI systems are transitioning towards the future. Given the vast number of AI papers in the literature, we first performed a search using the ISI Web of Knowledge on papers having one of the six AI-related terms (i.e., AI, machine learning, deep learning, data analytics, evolutionary algorithm and decision tree) and the word “water” in the title and then applied a snowballing method to identify the relevant papers in the references of previously identified papers. As a result, a total of 3,000 references were found from the literature search but following a careful review 85 references were selected for synthesis. A high proportion of references were excluded mainly due to (1) irrelevant application areas such as river basin water management, agriculture and hydrology, and (2) the same problems with different AI approaches. Note that this review is not intended to provide a comprehensive review of AI technologies and their application to specific water management problems as such reviews can be found in the literature (e.g., Eggimann et al., Reference Eggimann, Mutzner, Wani, Schneider, Spuhler, Moy De Vitry, Beutler and Maurer2017; Stewart et al., Reference Stewart, Nguyen, Beal, Zhang, Sahin, Bertone, Silva-Vieira, Castelletti, Cominola, Giuliani, Giurco, Blumenstein, Turner, Liu, Kenway, Savic, Makropoulos and Kossieris2018; Zounemat-Kermani et al., Reference Zounemat-Kermani, Matta, Cominola, Xia, Zhang, Liang and Hinkelmann2020; Fu et al., Reference Fu, Jin, Sun, Yuan and Butler2022; Garzón et al., Reference Garzón, Kapelan, Langeveld and Taormina2022).
How can AI improve urban water system performance?
This section first provides a general introduction of AI domains and their application to UWI systems, then explains the three categories of UWI system performance, and finally shows how the system performance could be improved via different types of AI analytics.
Types of AI and their applications to UWI systems
The definition and taxonomy of AI and its applications can vary widely across application areas. In this paper, we adopt the Joint Research Centre taxonomy (Samoili et al., Reference Samoili, Cobo, Gómez, De Prato, Martínez-Plumed and Delipetrev2020) which provides a useful reference for AI development, uptake and impacts. This AI taxonomy covers a wide range of core AI sub-domains and transversal areas. For the purpose of this paper, we do not include the transversal AI areas such as robots, AI services and philosophical considerations, which go beyond our direct aim of linking AI with UWI systems. The core AI can be divided into reasoning, planning, learning, communication and perception (Table 1). From the literature search, AI has a long history of intersecting urban water research, particularly in the area of planning, scheduling, optimisation and searching. In Table 1, we have shown examples of water applications that set out operation scheduling or optimise capacity expansion of urban water systems. Other AI domains of machine learning, natural language processing, computer vision and audio processing also present in water applications, such as in anomaly detection, water demand forecasting, flow forecasting, weather prediction, as well as other general water monitoring purposes (e.g., Eggimann et al., Reference Eggimann, Mutzner, Wani, Schneider, Spuhler, Moy De Vitry, Beutler and Maurer2017; Garrido-Baserba et al., Reference Garrido-Baserba, Corominas, Cortés, Rosso and Poch2020; Fu et al., Reference Fu, Jin, Sun, Yuan and Butler2022; Garzón et al., Reference Garzón, Kapelan, Langeveld and Taormina2022). To date, the AI domain of reasoning has not been as popular as the other fields in water application. However, its application is emerging due to the need for explicit reasoning, causality and explainability in AI water applications. It should be noted that the AI sub-domains are related and not disjoint (Samoili et al., Reference Samoili, Cobo, Gómez, De Prato, Martínez-Plumed and Delipetrev2020), consequently their applications to UWI systems are intertwined. For example, water distribution system design could use knowledge presentation to embed design rules in the optimisation process and integrate machine learning as a surrogate model to improve optimisation efficiency.
Table 1. The joint research centre AI taxonomy and relevant applications for UWI
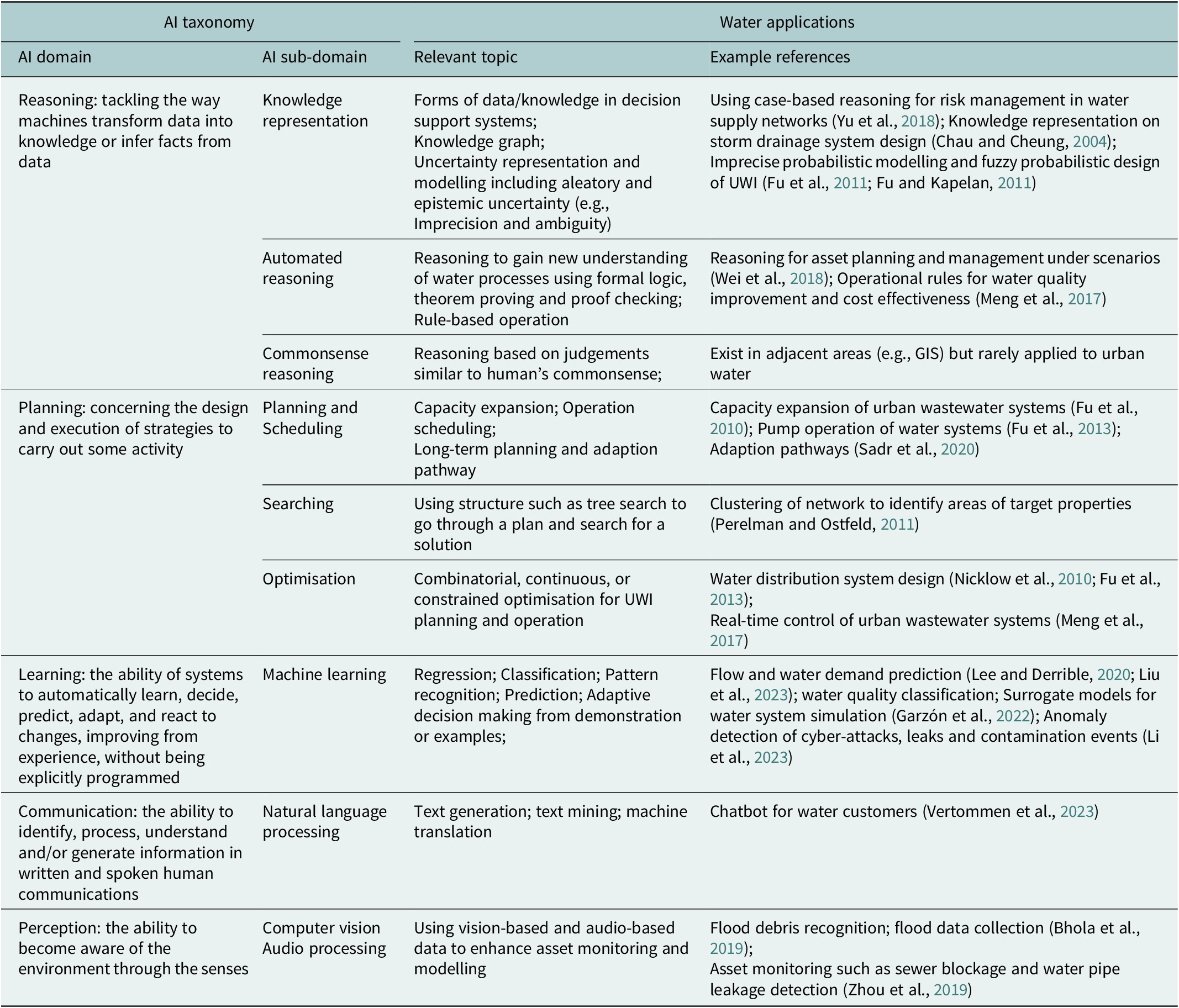
Reliability, resilience and sustainability
The performance of UWI systems can be broadly described within three categories: reliability, resilience and sustainability, which jointly describe the short- and long-term service level under various conditions. These categories are not mutually exclusive but form a pyramidal relationship: reliability is necessary but not sufficient for resilience, and resilience is necessary but not sufficient for sustainability (Casal-Campos et al., Reference Casal-Campos, Fu, Butler and Moore2015; Butler et al., Reference Butler, Ward, Sweetapple, Astaraie-Imani, Diao, Farmani and Fu2017). With each category, a wide range of metrics are available to measure the operational performance of each type of UWI systems, and they need to meet some specified goals to be classified as reliable, resilient or sustainable. However, difficulties normally arise from identifying which system properties contribute to achieving reliability, resilience and sustainability. AI can be a key tool to understand the complexity of UWI systems and their short- and long-term performance and then provide solutions for performance and capacity enhancement. In general, all the performance categories can be improved by four types of data analytics using AI approaches, referred to as AI analytics: descriptive, diagnostic, predictive and prescriptive analytics. A mapping between performance and AI analytics is provided in Figure 1 and explained in detail below.

Figure 1. Use of four AI analytics to improve urban water infrastructure performance.
Reliability has been at the heart of water system design for centuries and is normally defined to measure the system performance under standard loading conditions or known threats (Butler et al., Reference Butler, Ward, Sweetapple, Astaraie-Imani, Diao, Farmani and Fu2017). It is generally represented as a probabilistic term to consider stochastic uncertainties in loadings. Reliability-based design and operation aim to reduce the likelihood of failure to achieve the specified level of service as far as is cost-effectively possible but do not consider what happens when failure occurs. Risk, a closely related concept to reliability, incorporates the consequences (often in monetary terms) of system failure or system vulnerability, though risk is sometimes treated as equivalent to reliability in some contexts such as water quality or water supply risks (e.g., Borgomeo et al., Reference Borgomeo, Mortazavi-Naeini, Hall, O’Sullivan and Watson2016).
Resilience, a concept originally emerged from ecology in the 1970s, has long been applied to water system management (e.g., Hashimoto et al., Reference Hashimoto, Stedinger and Loucks1982). There are many definitions of water system resilience (Juan-García et al., Reference Juan-García, Butler, Comas, Darch, Sweetapple, Thornton and Corominas2017), but most emphasise the ability to cope with and recover from unexpected, disruptive events. In operation, resilience is normally measured by failure duration, failure magnitude, time to failure, speed of recovery or their combinations, so AI-based identification and characterisation of failures is crucial for understanding failure patterns and developing solutions to improve UWI resilience.
Sustainability measures the performance of UWI systems in economic, environmental and social dimensions (Vinuesa et al., Reference Vinuesa, Azizpour, Leite, Balaam, Dignum, Domisch, Felländer, Langhans, Tegmark and Fuso Nerini2020). A vast number of indicators are used for each dimension, for example: using capital and operational costs, life cycle costs, and energy consumption for the economic dimension; using greenhouse gas (GHG) emissions, water quality, and ecosystem services for the environmental dimension; and using equity, equality, affordability, and public health for the social dimension. From a wider perspective, Vinuesa et al. (Reference Vinuesa, Azizpour, Leite, Balaam, Dignum, Domisch, Felländer, Langhans, Tegmark and Fuso Nerini2020) has shown that AI can have a positive impact on all eight targets for SDG6 – clean water and sanitation though it may have a negative impact on five targets.
The concepts of reliability, resilience and sustainability can be examined from two aspects: operational performance and system property. The performance aspect defines what is expected from the system in terms of service but does not describe what properties are required to deliver the performance (Butler et al., Reference Butler, Ward, Sweetapple, Astaraie-Imani, Diao, Farmani and Fu2017). System performance may arise from a number of system properties, but any one property does not guarantee a certain level of performance. For example, resilient water distribution systems normally have the following properties: robustness, flexibility, connectivity, redundancy, rapidity and resourcefulness. However, increasing connectivity does not necessarily lead to enhanced resilience because highly connected nodes may increase cascading effects of some specific failures. Further, a certain set of system properties can be related to more than one category of performance.
A wide range of interventions are available to improve reliability, resilience and sustainability of UWI systems. For example, decentralised treatment, green infrastructure (GI) and SCADA are adopted in UWI systems around the world. The interventions can be broadly organised in five development pathways: decentralisation, greening, circular economy, decarbonisation and automation. Section 3 provides a description of these pathways and how they can be enhanced by AI technologies.
Descriptive analytics
Descriptive analytics concern the use of real time or historical data to answer the question ‘what is happening’ or ‘what has happened’. This could be related to the operational states of UWI systems (e.g., water quality, flow, pressure and/or depth), key components (e.g., valves and pumps), or asset condition (e.g., structural deterioration and defect grade). These analytics provide an improved understanding of system characteristics, historical trends, operational performance and infrastructure conditions, thus can be used to develop monitoring strategies, intervention measures, benchmark standards and regulatory policies for reliability enhancement. For example, analysis of influent and effluent data from a large number of WWTPs provides an insight into treatment efficiency, energy efficiency and eco-efficiency and so provides a basis for system design benchmarking (Dong et al., Reference Dong, Zhang and Zeng2017; Longo et al., Reference Longo, Hospido, Lema and Mauricio-Iglesias2018).
Descriptive analytics can improve our knowledge on the spatio-temporal variability of external drivers to water systems and interdependencies with other systems, and thus help characterise uncertainties related to system design and operation. For example, numerous AI approaches have been used to investigate the key environmental, demographic and economic factors affecting water demand (Guo et al., Reference Guo, Liu, Wu, Li, Zhou and Zhu2018; Lee and Derrible, Reference Lee and Derrible2020; Smolak et al., Reference Smolak, Kasieczka, Fialkiewicz, Rohm, Siła-Nowicka and Kopańczyk2020; Zounemat-Kermani et al., Reference Zounemat-Kermani, Matta, Cominola, Xia, Zhang, Liang and Hinkelmann2020), aiming to support decisions on the design and operation of water distribution systems and improve hydraulic system reliability. Machine learning has been applied to generate high-resolution precipitation nowcasts by effectively capturing spatio-temporal correlations (Chen et al., Reference Chen, Cao, Ma and Zhang2020), which can potentially be applied to reduce the occurrence of urban flooding or combined sewer overflows (CSOs). Similarly, wastewater flow in sewer systems can be characterised using catchment and climate variables (Kerkez et al., Reference Kerkez, Gruden, Lewis, Montestruque, Quigley, Wong, Bedig, Kertesz, Braun, Cadwalader, Poresky and Pak2016; Lund et al., Reference Lund, Falk, Borup, Madsen and Steen Mikkelsen2018; Zhang et al., Reference Zhang, Li, Snowling, Siam and El-Dakhakhni2019) to improve operational reliability of WWTPs.
Diagnostic analytics
Diagnostic analytics are the examination of data to answer the question ‘why is it happening’ or ‘why did it happen’. It can be used to tackle failure events related to key UWI components such as water mains, sewers, CSOs, pumps, valves, aerators and sensors. New knowledge on causal relationships and failure patterns derived from diagnostic analytics can help enhance system reliability through improved design and operation. Further, system resilience can be enhanced predominantly through rapid and accurate identification of failure events to reduce their social-environmental impacts.
In water distribution systems, the deployment of online leakage detection and decision support tools can help pinpoint leaks (Zhou et al., Reference Zhou, Tang, Xu, Meng, Chu, Xin and Fu2019; Zaman et al., Reference Zaman, Tiwari, Gupta and Sen2020; Wang et al., Reference Wang, Guo, Liu, Wu, Xu and Smith2020b) and contamination event detection (Arad et al., Reference Arad, Housh, Perelman and Ostfeld2013), and thus reduce the impacts of failure (Romano et al., Reference Romano, Kapelan and Savić2014; Nikoloudi et al., Reference Nikoloudi, Romano, Memon and Kapelan2021) through rapid interventions even before water services are affected. Machine learning can identify key factors of pipe failure related to infrastructure, operation and environmental factors, and thus help develop strategies for predictive maintenance (Barton et al., Reference Barton, Hallett and Jude2022). Machine learning can significantly improve leak localisation accuracy, even with a small amount of newly monitored data when implemented real-time (Zhou et al., Reference Zhou, Tang, Xu, Meng, Chu, Xin and Fu2019). Most importantly, machine learning is the predominating approach to solve the leakage detection problem as other approaches such as mechanism models are limited due to lack of understanding of pipe deterioration mechanisms.
Similar problems in the sewer system include blockage detection and localisation (Ugarelli et al., Reference Ugarelli, Venkatesh, Brattebø, Di Federico and Sægrov2010; Okwori et al., Reference Okwori, Viklander and Hedström2021), CSO analysis and source apportionment of water pollution (Huang et al., Reference Huang, Wang, Lou, Zhou and Wu2010; Chen et al., Reference Chen, Qin, Zheng and Fu2019), and infiltration/exfiltration identification and localisation (Karpf and Krebs, Reference Karpf and Krebs2011; Guérineau et al., Reference Guérineau, Dorner, Carrière, McQuaid, Sauvé, Aboulfadl, Hajj-Mohamad and Prévost2014). Diagnostic analytics can be used to identify sensor failures such as sensor reading drift using long-term continuous monitoring (Wang et al., Reference Wang, Fan, Huang, Ling, Klimowicz, Pagano and Li2020a). In wastewater treatment processes, system faults and condition changes can be detected using statistical process control-based methods considering single or multiple variables (Newhart et al., Reference Newhart, Holloway, Hering and Cath2019).
A holistic understanding of cause-effect relationships concerning failure events is useful for UWI design and retrofit processes as resilience-enhancing properties derived by data analytics can be incorporated. For example, the interplay between six key topological attributes (i.e., connectivity, efficiency, centrality, diversity, robustness and modularity) and six resilience metrics related to system resistance, absorption and restoration capacities can guide the re-design of water distribution systems to make them more resilient (Meng et al., Reference Meng, Fu, Farmani, Sweetapple and Butler2018).
Predictive analytics
Predictive analytics are the use of real time or historical data to answer the question ‘what will or is likely to happen’. In addition to physics-based models, a wide range of data-driven approaches have been reported to predict future states, performances, events and asset conditions in UWI systems. One key aspect of sustainability involves the use of predictive analytics to assess the impacts of interventions and operations, because sustainability concerns the long-term performance of water systems, in some situations, beyond their design life. Predictive analytics can be used to estimate when key components of the system are likely to fail and thus plan maintenance work accordingly to save costly repairs or reduce extended downtime periods, which is referred to as predictive maintenance. One example of predictive maintenance is pipe deterioration prediction using AI approaches for pipe condition assessment in support of maintenance investment decisions (Berardi et al., Reference Berardi, Giustolisi, Kapelan and Savic2008; Scheidegger et al., Reference Scheidegger, Leitão and Scholten2015).
The improvement of system performance can be materialised through design and retrofit solutions, control strategies, operational actions, emergency plans and long-term adaption strategies generated from prescriptive analytics, which is normally built on the insight and knowledge gained from the other types of analytics. It is important to stress that reliability, resilience and sustainability are interlinked, the three types of data analytics, that is, descriptive, diagnostic and predictive, can provide new knowledge on the system which is then used by prescriptive analytics for actions.
Prescriptive analytics
Prescriptive analytics answers the question ‘how to operate or manage’, normally based on causal relationships and predicted outcomes using the other AI analytics. It can be used to recommend optimal operation and control strategies, real-time scheduling, and design and retrofit solutions, intervention measures and long-term management strategies in the life cycle of UWI systems. This type of analytics often refers to optimisation-based approaches. Evolutionary algorithms have become a flexible, powerful and well-used tool in solving complex water problems and applied to provide optimal operation and management options for UWI systems (Nicklow et al., Reference Nicklow, Reed, Savic, Dessalegne, Harrell, Chan-Hilton, Karamouz, Minsker, Ostfeld, Singh and Zechman2010; Maier et al., Reference Maier, Kapelan, Kasprzyk, Kollat, Matott, Cunha, Dandy, Gibbs, Keedwell, Marchi, Ostfeld, Savic, Solomatine, Vrugt, Zecchin, Minsker and Barbour2014). Though most machine-learning algorithms are generally used to gain insights from data and are not suitable for solving optimisation problems, they can be used as surrogate models in the optimisation process to improve search efficiency (Garzón et al., Reference Garzón, Kapelan, Langeveld and Taormina2022). However, reinforcement learning, a nascent type of machine learning, is able to solve sequential planning and operation problems and has been used to develop control strategies for urban flood and WWTP operation problems (Fu et al., Reference Fu, Jin, Sun, Yuan and Butler2022).
It should be noted that the four types of analytics are closely linked and sometimes a combination of different types are used to solve a practical water problem. For example, in addressing leakage and contamination detection problems, descriptive and predictive analytics can be used to understand the historical system behaviour and predict the system state respectively, and then the residuals between predicted and observed state variables are used to identify anomalies through comparison with the system variability under historical normal conditions (Arad et al., Reference Arad, Housh, Perelman and Ostfeld2013; Wang et al., Reference Wang, Guo, Liu, Wu, Xu and Smith2020b). When developing operational actions using prescriptive analytics, predictive analytics is used to estimate future system states so actions can be developed considering expected outcomes.
Moving from descriptive to prescriptive analytics increases the autonomy and intelligence of UWI systems while increasing the difficulty in the development of algorithms. All four types transform data into information, insight and knowledge, but only prescriptive analytics is able to make ‘decisions’ by generating new solutions, actions and interventions, thus moving from dumb interventions to near-optimal interventions. With the advance of wireless sensors, universal connectivity, high-speed data analytics and AI technologies, the possibility of transforming existing UWI systems into autonomous systems becomes increasingly realistic. With an autonomous water system, the degree of human intervention is low and the system is capable of performing a series of optimal operations in response to real-time, dynamic environments that are monitored and measured within the system itself.
How can AI shape the future of urban water infrastructure?
Five key characteristics of future UWI are envisioned as decentralised, green, circular, carbon neutral, and autonomous, based on the gradual evolution of water systems through interventions implemented in the last three decades. The development pathways to these characteristics are not mutually exclusive but interconnected in terms of enhancing system performance, capacity and efficiency, which ultimately contribute to resilient and sustainable water systems. For example, although adoption of swales for stormwater management is an approach to the greening pathway, it could be part of decarbonisation and decentralisation pathways as it can reduce energy consumption and GHG emissions and de-connect stormwater services from centralised sewer systems. AI technology could be incorporated in the planning, design, operation and management processes of UWI systems, as discussed in Section 2; thus, it underpins all the five development pathways: decentralisation, circular economy, decarbonisation, greening, and automation (Figure 2).

Figure 2. Five pathways towards more reliable, sustainable and resilient urban water infrastructure underpinned by artificial intelligence.
Decentralisation pathway
AI can play a key role in planning and management of decentralised systems where local facilities are optimised for water supply, stormwater management, water recycling and wastewater treatment to avoid unnecessary loss of resources (i.e., water, energy and materials). Compared to centralised systems, optimal planning of decentralised systems is a more complex problem as they should be designed to link individual decentralised systems into a system of systems that functions as a whole to achieve the required water and wastewater services and maximum environmental and ecological benefits. On transitioning to the future system, the degree of decentralisation is a key design factor for integrating decentralised systems into the existing UWI configuration and it could be optimised with prescriptive analytics such as evolutionary algorithms, considering economies of scale, topographical structure, technological advances and operational efficiency (Eggimann et al., Reference Eggimann, Truffer and Maurer2015).
Decentralisation is primarily driven by technological advances in on-site water and wastewater treatment and recycling. Garrido-Baserba et al. (Reference Garrido-Baserba, Barnosell, Molinos-Senante, Sedlak, Rabaey, Schraa, Verdaguer, Rosso and Poch2022) suggested that existing technologies can be used to create economically viable decentralised systems that eliminate the need for centralised water supply or sewage collection under some conditions. However, AI technologies are required to maximise multiple benefits of individual decentralised systems through identification of reliable and resilient operations and development of predictive maintenance strategies. In particular, AI could be used to facilitate surveys that are designed to understand public perceptions of decentralised systems by identifying participants, targeting a certain group of people and analysing collected data, and it has been increasingly used in citizen science projects for better engagement and task allocation (Ponti and Seredko, Reference Ponti and Seredko2022). This could help develop climate-resilient solutions for wider-adoption.
Circular economy pathway
WWTPs are at the heart of the circular economy approach as they are not only energy-intensive accounting for 25% of the total energy use in the water sector, but also central for water reuse, renewable energy generation and nutrient recovery with a range of technologies available (Rani et al., Reference Rani, Snyder, Kim, Lei and Pan2022). Process-based models have been used for optimising energy and nutrient recovery from wastewater treatment processes (Nakkasunchi et al., Reference Nakkasunchi, Hewitt, Zoppi and Brandoni2021). In response to a particular real-world challenge in highly variable wastewater streams, real-time monitoring of critical process parameters and nutrient attributes is increasingly implemented. This allows an integration of machine learning for accurate system dynamics simulation, enabling online learning and real-time control for maximum resources recovery.
Integration of water supply, stormwater, and wastewater systems is critical in re-shaping the flows of water, energy and materials in a UWI system to maximise reuse, recycling and recovery of resources where possible given dynamic demands and disturbances. Grey water is increasingly used for potable and non-potable water supplies, however, AI could play a key role in real-time monitoring and detection of any changes in water quality due to pathogens or chemical pollutants and facilitate rapid and appropriate responses to alleviating public health concerns and widening the adoption in communities.
The complex interrelations between various components in the UWI system provides the potential of building a water-energy-materials nexus to boost the adoption of the circular economy approach in urban water management (Fu and Butler, Reference Fu, Butler, Wang and Fu2021). AI approaches could predict future demands in water and energy and optimise resource efficiency across the entire system where individual components are operated jointly to common goals. For example, a large increase in intermittent renewable energy sources could be integrated seamlessly with the operation of energy-intensive water reuse facilities to achieve energy positive while ensuring stable and reliable operation.
Greening pathway
The greening development pathway practised in the water sector through the concept of urban GI represents a nature-based approach to stormwater and flood management. It is now widely accepted as part of UWI systems and implemented with various measures (e.g., green roofs, swales, bio-retention ponds) to achieve a wide range of benefits in water security, flood management, urban pollution, ecosystem services, carbon reduction, public health and well-being in different cities (Oral et al., Reference Oral, Carvalho, Gajewska, Ursino, Masi, Hullebusch, Kazak, Exposito, Cipolletta, Andersen, Finger, Simperler, Regelsberger, Rous, Radinja, Buttiglieri, Krzeminski, Rizzo, Dehghanian, Nikolova and Zimmermann2020). GI planning will benefit from spatial data analytics to provide insights in their impacts on system resilience, flood mitigation, and water security, ensuring GI an integral part of UWI systems as a major challenge in GI application is its design as single entities (Kerkez et al., Reference Kerkez, Gruden, Lewis, Montestruque, Quigley, Wong, Bedig, Kertesz, Braun, Cadwalader, Poresky and Pak2016).
A key challenge in promoting GI is the significant functional impairment in practice due to inappropriate operation and lack of maintenance over time. In-situ performance metrics of GI can be provided by AI tools based on continuous monitoring of water flow, water level, soil-moisture, and water quality. This information can be further fed into real-time control of stormwater systems and decision-making of retrofitting and predictive maintenance. Rainwater harvesting systems (e.g., water butts) can be operated to reduce flood risk based on rainfall forecasts provided by machine learning, with positive community engagement to emptying water before predicted rainfall events.
Sensor networks deployed for various purposes (e.g., water quality, air quality, temperature, sound and light) in a smart city should be integrated to expand our understanding of spatio-temporal ecosystem services provided by GI and thus identify prioritised interventions. Biotic and anthropogenic acoustic activity, predicted by deep learning from audio recordings provides new understanding of urban soundscapes and facilitate investigation of the impact of GI on wildlife (Fairbrass et al., Reference Fairbrass, Firman, Williams, Brostow, Titheridge and Jones2019). Urban heat island effects can be reduced by effective planning of green roofs while tackling stormwater and urban runoff pollution problems. The growing evidence on multiple benefits of GI can further stimulate the desire for GI in lieu of conventional grey infrastructure (Oral et al., Reference Oral, Carvalho, Gajewska, Ursino, Masi, Hullebusch, Kazak, Exposito, Cipolletta, Andersen, Finger, Simperler, Regelsberger, Rous, Radinja, Buttiglieri, Krzeminski, Rizzo, Dehghanian, Nikolova and Zimmermann2020).
Decarbonisation pathway
Recent studies have highlighted the importance of GHG emissions from water-related activities and the vital role of the water sector in climate change mitigation (Rothausen and Conway, Reference Rothausen and Conway2011; Rani et al., Reference Rani, Snyder, Kim, Lei and Pan2022). Energy consumption and wastewater treatment are major sources of GHG emissions in the water sector. Although new technologies have been deployed to increase renewables generation, increase energy efficiency and reduce emissions from wastewater treatment with an aim to achieving net zero targets in the water sector, the role of AI in achieving net zero emissions is increasingly recognised, as shown by the UK’s AI roadmap (UK AI COUNCIL, 2021).
Monitoring of GHG emissions in the UWI is a challenge facing the water sector, mainly due to highly complex and site-specific production processes of nitrous oxide and methane emissions and high costs of sensors. Machine learning has already shown promise in designing monitoring schemes and estimating GHG emissions in WWTPs, which help develop monitoring guidelines (Ye et al., Reference Ye, Porro and Nopens2022). A machine-learning model trained with full-scale data can be used as soft sensors to provide continuous GHG emissions data. This is valuable in providing insights into GHG emissions variability or production processes by integrating soft sensors and mechanistic models (Li et al., Reference Li, Duan, Liu, Qiu, van den Akker, Ni, Chen, Yin, Yuan and Ye2022b).
Optimal control empowered by AI can reduce both direct and indirect GHG emissions in the UWI system. WWTPs are highly energy-dependent, so control strategies could be developed using a range of AI methods to balance the GHG emissions and effluent water quality (Sweetapple et al., Reference Sweetapple, Fu and Butler2014), though a positive correlation between nitrous oxide emissions and total nitrogen is commonly accepted (Duan et al., Reference Duan, van den Akker, Thwaites, Peng, Herman, Pan, Ni, Watt, Yuan and Ye2020). Pump scheduling in water distribution and wastewater systems should consider future demand or flow predictions, intermittence of on-site renewables and varying prices of the grid energy. Aeration pumping can be optimised to make use of varying dilution capacity in the receiving water while meeting water quality standards (Meng et al., Reference Meng, Fu and Butler2017).
Automation pathway
Automation is widely utilised in water and WWTPs due to advances in sensing, communication and computing technologies, however, it is mainly limited to control of individual process units or several units in a system (Yuan et al., Reference Yuan, Olsson, Cardell-Oliver, van Schagen, Marchi, Deletic, Urich, Rauch, Liu and Jiang2019). Research on integrated control of UWI systems has progressed rapidly over the past decades (Fu et al., Reference Fu, Butler and Khu2008), however real-world applications are limited. Challenges in improving automation or building fully autonomous water systems are profound from many aspects: complex interactions between different system components, uncertainties in future predictions, explainability of control strategies and AI trustworthiness, capital investments required for monitoring and software.
Machine learning can be integrated into system control methods for improved control performance. Significantly improved water quality, system reliability and energy efficiency are amongst the benefits reported through AI integration, for example, the use of forecasted demands to control the outflow from water treatment plants (Bakker et al., Reference Bakker, Vreeburg, Palmen, Sperber, Bakker and Rietveld2013) and the use of rainfall and flow forecasts to control CSOs and wastewater treatment processes (Lund et al., Reference Lund, Borup, Madsen, Mark, Arnbjerg-Nielsen and Mikkelsen2019). The latest deep learning technology has greatly improved the forecasting accuracy in water demand, pressure, flow, water depth and pollution load (Wang et al., Reference Wang, Man, Hu, Li, Hong and Cui2019), thus is promising in improving system control.
Deep reinforcement learning has great promise of tackling the problems in current control algorithms such as highly uncertain disturbances and intensive computing times. It is anticipated that research in deep reinforcement learning in the next decades will enable more automated control of the UWI system or the incorporation of some autonomous components into physical infrastructure systems.
AI attributes to support development pathways
AI has many attributes that distinguish it from other technologies. The following key attributes are well suited to support the development pathways identified and should be considered when designing an AI system to solve water management problems.
-
1. Adaptability. AI systems can adapt to changing conditions and environments and improve their performance over time without explicit programming. This is mainly achieved through machine learning to allow self-learning from data and experience. This attribute is useful for accurate representation of UWI systems, which often change in response to urbanisation and climate change through engineering intervention.
-
2. Autonomy: AI systems can be designed to operate independently with no or low level of direct human supervision. Many repetitive tasks in the management of UWI systems could be potentially automated at relatively low-cost (Garrido-Baserba et al., Reference Garrido-Baserba, Corominas, Cortés, Rosso and Poch2020). One example is sewer defect detection through computer vision technologies to process large amounts of CCTV images obtained by water companies (Myrans et al., Reference Myrans, Everson and Kapelan2018).
-
3. Scalability: AI systems can be easily scaled up or down to meet the needs of different water systems and data availability. They can be developed and deployed for a component, decentralised system at household and community levels, or large-scale system at the city and catchment levels. In particular, they are capable to handle large amounts of data, making it suitable for large-scale systems.
-
4. Parallellisation: AI systems can make use of parallel computing resources and distributed data for efficient calculation and effective communication. They could be used for integrated control of a water system, where the operation of individual controllers is based on independently trained AI systems to capture local conditions but co-ordinated across the system to achieve high-level system objectives. For example, multiple agents have been developed to optimise DO and dosage simultaneously in wastewater treatment processes (Chen et al., Reference Chen, Wang, Valverde-P’erez, Zhai, Vezzaro and Wang2021).
-
5. Efficiency: AI systems can be computationally efficient in handling large amounts of data and large-scale systems. Though many machine-learning algorithms could be time-consuming during training, they are extremely efficient in implementation after training. Further recent advances have been made in data-centric approaches which explore training with small data sets (Liu et al., Reference Liu, Savic and Fu2023), and transfer learning which allow algorithms to perform different tasks which they are not trained for. This makes AI systems suitable as a surrogate of hydraulic models for computationally intensive tasks such as optimal system design, real-time control, and uncertainty analysis (Garzón et al., Reference Garzón, Kapelan, Langeveld and Taormina2022).
The AI attributes have been translated into advantages as shown by case studies in the literature (Fu et al., Reference Fu, Jin, Sun, Yuan and Butler2022). Examples include processing high spatio-temporal resolution data for demand prediction (Zounemat-Kermani et al., Reference Zounemat-Kermani, Matta, Cominola, Xia, Zhang, Liang and Hinkelmann2020), considering correlations of multi-variate time series for more accurate contamination detection (Li et al., Reference Li, Liu, Zhang and Fu2023), high efficiency and accuracy in video processing (Myrans et al., Reference Myrans, Everson and Kapelan2018), and robust control in an uncertain environment (Xu et al., Reference Xu, Meng, Guo, Li and Fu2021b).
Practical implications and barriers in AI application
Although the transformative potential of AI in UWI becomes clear in enhancing performance, capacity and efficiency, we must recognise a number of practical implications and barriers may that limit its wide adoption in the water sector to support the five pathways described. The implication and barriers can be categorised in terms of four dimensions: cyber-physical infrastructure and institutional governance in the water sector, and social-economic and technological development in wider society, as shown in Figure 3.

Figure 3. Practical implications and barriers for AI adoption in the water sector.
Public sector water utilities generally lack a clear strategy for digital transformation and organisational culture for changes in technology and operations as they do not face competitive market pressures, nor do they have the option of rapidly developing new business models (Boyle et al., Reference Boyle, Ryan, Bhandari, Pratik, KMY, Gong and Creighton2022). This leads to low investments for development of digital skills in the water sector. A healthy AI ecosystem involving water utilities, IT companies, consultancies and academia is needed to speed up the adoption of AI tools for water management (Garrido-Baserba et al., Reference Garrido-Baserba, Corominas, Cortés, Rosso and Poch2020).
Given the complexity of UWI systems, difficulties remain in identifying AI applications which can make a breakthrough in fundamentally transforming water system operation and management, though a few areas such sewer defect and leakage detection are emerging (Fu et al., Reference Fu, Jin, Sun, Yuan and Butler2022). Development of tailor-made AI models based on the latest deep learning algorithms might improve the availability and maturity of AI technology in the water sector. Availability of large data sets should be tackled when identifying and developing AI tools. Improving explainability is also key for users to understand predictions and decisions from AI tools and thus encourage their adoption in the water sector.
Though the advance in information and communication technologies has enabled the proliferation of sensors in UWI systems, there is still a significant gap in system monitoring considering the large number of variables and distributed components that need monitoring. Research communities continue to develop low cost and high reliability sensors required for understanding real-time system dynamics. Significant capital investment is needed for data infrastructure, AI platforms and tools that can store and process the unprecedented amount of data being collected.
When AI becomes embedded in many processes, services and products in the water sector, it will have a profound impact on society from many aspects: data privacy, ethical and legal concerns, risks and unintended consequences from adoption of AI-based systems and their failure (de Vitry et al., Reference de Vitry, Schneider, Wani, Manny, Leitao and Eggimann2019). Some fear that automation driven by AI could lead to job losses. Incorporating AI to support the pathways needs to reflect its speed and scope of adoption, so appropriate regulation and governance is needed to enable accountability, trustworthiness and transparency to ensure social and economic benefits from AI research and development.
Conclusions
This review sought to analyse and discuss how AI might shape the future development of UWI systems. The four types of AI analytics, descriptive, diagnostic, predictive and prescriptive, are found to contribute to improving system performance based on three categories, that is, reliability, resilience and sustainability. We envision a future where AI will support the development of five pathways towards UWI systems of the future: decentralisation, circular economy, greening, decarbonisation and automation. This shows profound opportunities of AI application for the water sector in terms of how interventions should be developed and incorporated into the existing infrastructure system using AI-based methods and tools. The barriers and challenges in AI adoption for enhancing the development of pathways are characterised on the basis of four dimensions: cyber-physical infrastructure, institutional governance, social-economic systems and technological development in the water sector and wider society.
We advocate a pivotal role of AI technologies in moving towards reliable, resilient and sustainable UWI systems. Given the breadth and significance of potential AI applications, the water sector needs to significantly increase capital investments on AI systems and skills, building on the investments already made to SCADA and digitalisation in the last three decades. Developing an interdisciplinary and interactive approach to tackling the barriers is essential to ensure that AI-based systems are developed and deployed in a responsible way to build the future UWI systems.
The synthesis of the role of AI was focused on the cyber-physical component of UWI in this review. In addition, AI could support the social-economic aspects of UWI, such as public engagement, public perception, human–infrastructure interactions, citizen science and customer service, which should be further analysed in the future.
Open peer review
To view the open peer review materials for this article, please visit http://doi.org/10.1017/wat.2023.15.
Data availability statement
Data sharing are not applicable to this article as no datasets were generated during the current study.
Author contribution
All authors contributed to the conceptualisation and development of this manuscript. G.F. wrote the initial draft and all authors reviewed, edited and approved the final manuscript.
Financial support
This work was supported by the Royal Society under the Industry fellowship scheme (grant number IF160108); the Alan Turing Institute under the EPSRC grant (grant number EP/N510129/1); and the National Natural Science Foundation of China (grant number 42071272).
Competing interest
There are no financial or nonfinancial interests to disclose by the authors.
Comments
No accompanying comment.